题目:Harmonic Mean Iteratively Reweighted Least Squares for Low-Rank Matrix Recovery 报告人:Christian Kümmerle 时间:2017年9月5日星期二,晚上18:00到20:00 地点:格物楼518 摘要:We propose a new iteratively reweighted least squares (IRLS) algorithm for the recovery of a matrix X in dimension (d_1 x d_2) of rank r from incomplete linear observations, solving a sequence of low complexity linear problems. The easily implementable algorithm, which we call harmonic mean iteratively reweighted least squares (HM-IRLS), optimizes a non-convex Schatten-pp quasi-norm penalization to promote low-rankness and carries three major strengths, in particular for the matrix completion setting. First, the algorithm converges globally to the low-rank matrix for relevant, interesting cases, for which any other (non-)convex state-of-the-art optimization approach fails the recovery. Secondly, HM-IRLS exhibits an empirical recovery probability close to 1 even for a number of measurements very close to the theoretical lower bound r(d1+d2−r),i.e., already for significantly fewer linear observations than any other tractable approach in the literature. Thirdly, HM-IRLS exhibits a locally superlinear rate of convergence (of order 2−p) if the linear observations fulfill a suitable null space property. While for the first two properties we have so far only strong empirical evidence, we prove the third property as our main theoretical result.Joint work with Juliane Sigl (Technische Universität München, Germany). 个人简介:Christian Kümmerle是德国慕尼黑工业大学博士,曾在法国巴黎第六大学和美国弗吉尼亚大学交流。他的研究兴趣方向是统计、最优化、高维概率理论的交叉研究和它们在数据相关问题中的应用。 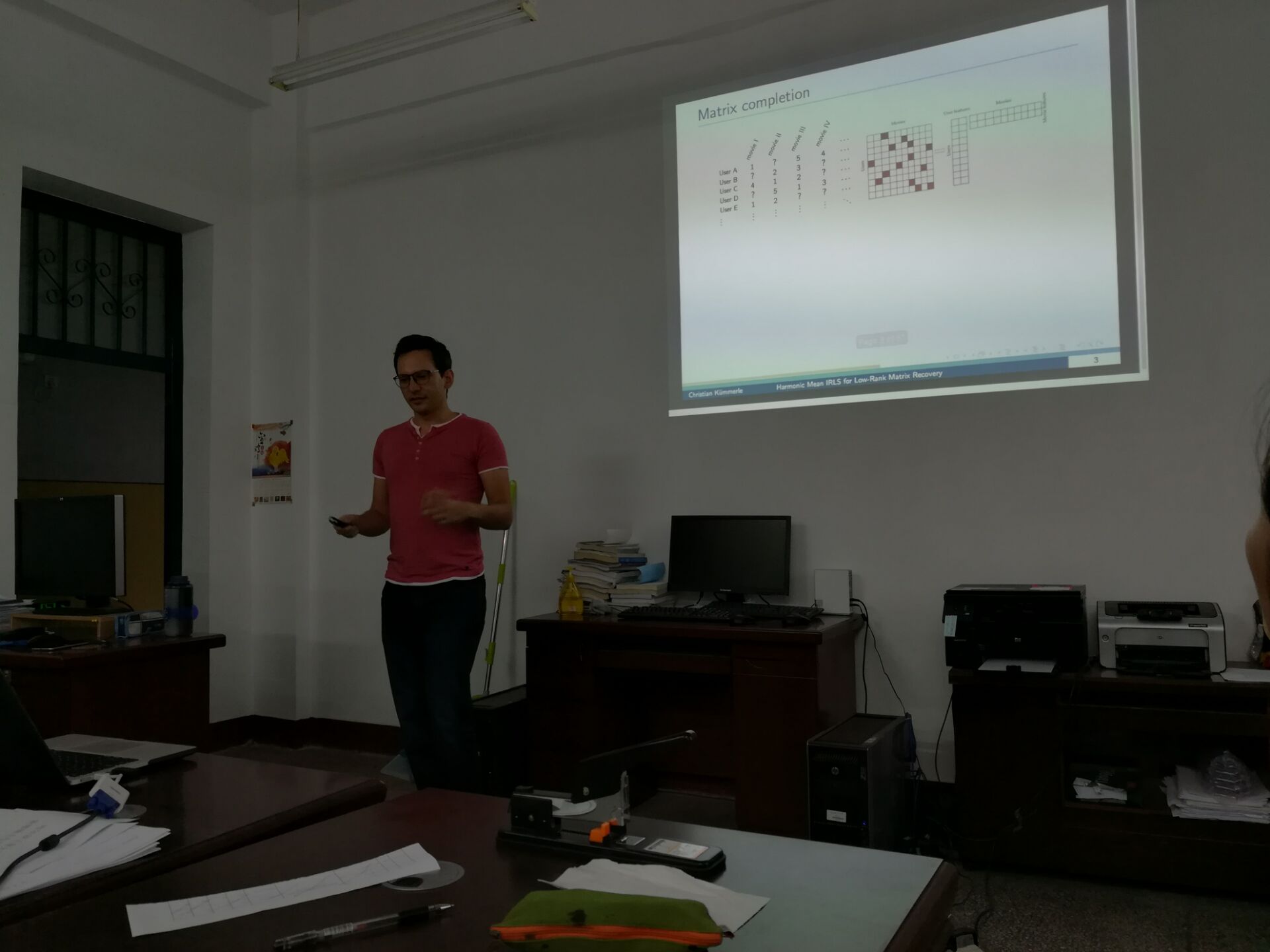
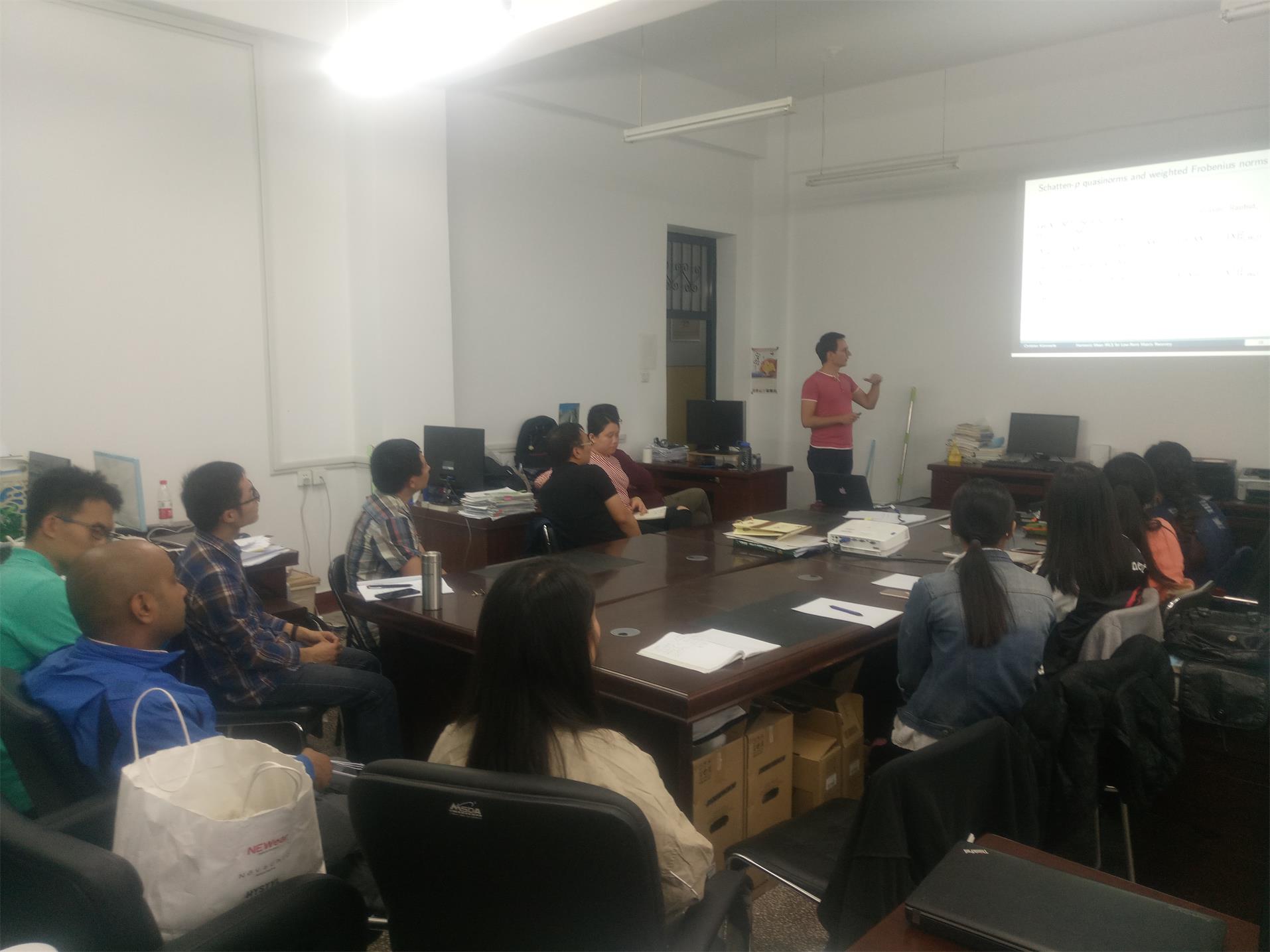 |